Implementing AI for Predictive Maintenance in Electrical Systems
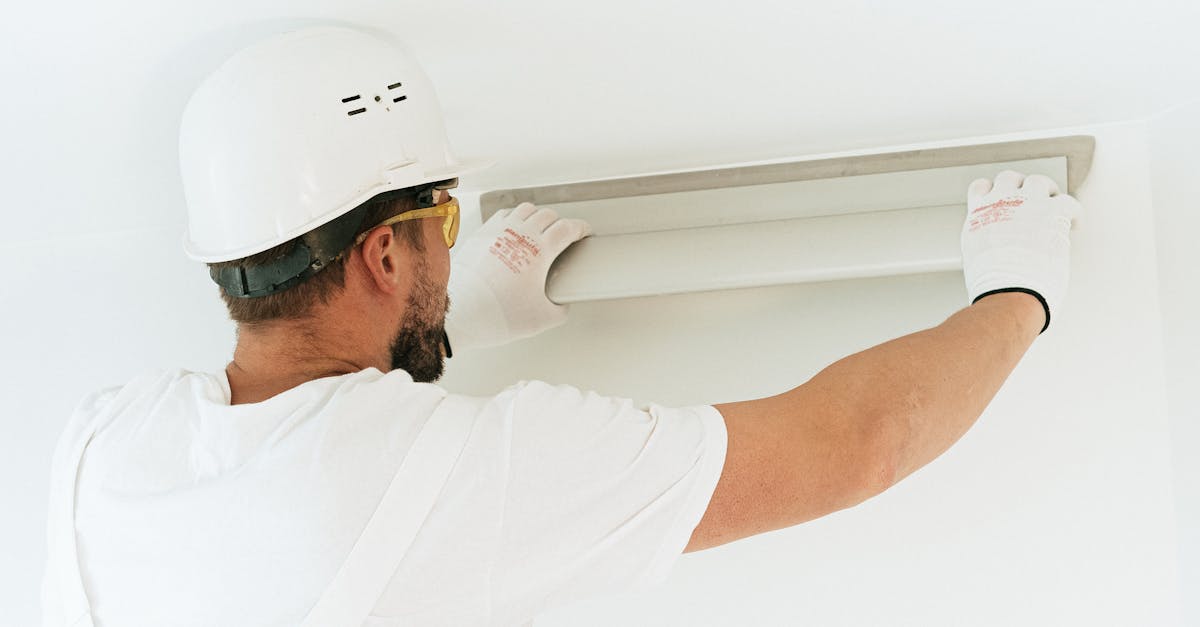
Table Of Contents
Implementing AI Solutions
Incorporating artificial intelligence into predictive maintenance requires a systematic approach. The initial step involves assessing the existing electrical systems to identify areas where AI technology can add value. This evaluation should include a thorough analysis of historical performance data and maintenance records. Understanding the specific needs and pain points of the system helps tailor the AI solution effectively. Gathering data from various sources fosters a comprehensive dataset for training AI algorithms, enhancing their predictive capabilities.
Following the assessment, the next phase focuses on selecting the appropriate AI tools and platforms. Several options are available, from machine learning models to advanced analytics software. Collaboration with technology providers can further streamline the integration process, ensuring that the chosen solutions are compatible with existing infrastructure. Rigorous testing and validation of the AI system are critical to ensure accuracy and reliability in predictions. This process not only builds confidence among maintenance teams but also sets the foundation for effective implementation across the organisation.
Steps for Successful Deployment
A clear understanding of the existing infrastructure is vital before integrating AI solutions into electrical systems. Conducting a thorough assessment of the current equipment, data flow, and maintenance protocols helps identify areas where AI can provide the most value. Establishing a baseline for performance metrics is essential. This enables organisations to measure the effectiveness of AI-driven enhancements post-deployment. Stakeholders should ensure that all team members are aligned with the goals of the project.
Choosing the right AI tools and technologies is crucial for a successful outcome. A comprehensive evaluation of potential software platforms and hardware is necessary for compatibility with existing systems. Collaborating with technical experts can aid in selecting the most appropriate solutions tailored to the specific needs of the organisation. Once the tools are in place, necessary training sessions for staff should be planned. This fosters familiarity and confidence in using AI tools effectively for maintenance purposes.
Challenges in AI Implementation
The integration of AI into existing systems often encounters resistance from staff unfamiliar with new technologies. Employees may fear job displacement or lack confidence in using AI-driven tools. This cultural hurdle can result in reluctance to adopt innovative practices. Training and upskilling play a vital role in alleviating these concerns, fostering a more positive attitude towards AI.
Another significant challenge lies in data management and quality. AI algorithms rely on vast amounts of high-quality data to deliver accurate predictions. In many cases, organisations struggle with data silos, where information is trapped in separate systems. This fragmentation inhibits a comprehensive analysis necessary for effective AI deployment. Ensuring accessibility and consistency of data is crucial for overcoming this barrier and realising the full potential of AI in predictive maintenance.
Common Obstacles and Solutions
Organisations often face resistance from staff when integrating AI into existing maintenance processes. This hesitation stems from a fear of job displacement and a lack of understanding of AI capabilities. To address this, it is essential to communicate the benefits of AI, such as improved efficiency and the potential for upskilling employees in new technologies. Providing training sessions that focus on how AI can enhance rather than replace human work can help ease concerns and foster a more positive attitude toward the change.
Data quality remains a significant barrier during AI implementation. Inaccurate or incomplete data can lead to unreliable predictive models, undermining the effectiveness of AI solutions. To mitigate this, organisations should conduct thorough data audits before deployment. Investing in data cleaning and proper management processes will ensure that the foundation for AI analysis is solid. Additionally, collaboration with IT and analytics teams can play a crucial role in establishing best practices for data utilisation and maintenance.
Cost-Benefit Analysis of AI in Maintenance
Investing in AI for predictive maintenance involves a thorough evaluation of its potential return. Cost savings can stem from reduced downtime and extended equipment lifespan. Accurate predictions of maintenance needs help to optimally schedule servicing, minimising disruptions to operations. Additionally, AI systems can analyse vast datasets, identifying patterns and anomalies that traditional methods may overlook. This leads to improved decision-making and resource allocation.
The initial investment in AI technology and training may seem daunting. However, the long-term benefits often outweigh these costs. Enhanced operational efficiency can reduce overall maintenance expenses. Increased reliability and safety also contribute to a more productive work environment. Regular assessments of AI effectiveness will ensure that organisations maximally leverage their investments while adapting to evolving needs.
Evaluating ROI for Electrical Systems
The return on investment (ROI) for AI implementation in electrical systems often requires a comprehensive assessment of both tangible and intangible benefits. Factors such as reduced downtime, extended equipment lifespan, and enhanced safety protocols contribute to a more reliable operational environment. Quantifying the financial implications of these improvements involves measuring baseline performance metrics before AI integration. This approach enables businesses to track progress and attribute savings directly to AI-driven maintenance efforts.
In addition to direct cost savings, long-term operational efficiencies play a crucial role in evaluating ROI. By harnessing AI algorithms, companies can predict equipment failures more accurately, leading to timely repairs that prevent costly shutdowns. The integration of AI also fosters a culture of proactive maintenance, promoting the effective use of resources and energy. Understanding these facets allows organisations to make informed decisions about resource allocation and future investments in technology enhancements.
FAQS
What is predictive maintenance in electrical systems?
Predictive maintenance is a proactive approach that uses AI and data analytics to predict when equipment is likely to fail, allowing for timely maintenance and reducing unplanned downtime.
How can AI improve predictive maintenance?
AI enhances predictive maintenance by analysing vast amounts of data from electrical systems, identifying patterns, and providing insights that help predict potential failures more accurately than traditional methods.
What are the key steps for implementing AI solutions in predictive maintenance?
Key steps include defining objectives, collecting and organising data, selecting appropriate AI tools, training models, testing and validating outcomes, and integrating the solution into existing maintenance workflows.
What are some common challenges faced during AI implementation?
Common challenges include data quality issues, lack of skilled personnel, resistance to change within the organisation, and the integration of AI systems with existing infrastructure.
How can organisations evaluate the ROI of AI in maintenance?
Organisations can evaluate ROI by analysing cost savings from reduced downtime, improvements in maintenance efficiency, and the longevity of electrical systems, compared to the initial investment and operational costs of AI implementation.
Related Links
Utilising Drones for Electrical Infrastructure InspectionsIntegration of Smart Grids in Urban Electrical Networks
Innovations in Energy Management Software for Enterprises
Development of Augmented Reality Tools for Electricians
Trends in Modular Electrical Systems for Rapid Deployment
Advancements in Solar Technology for Commercial Applications